AI and Cancer Research
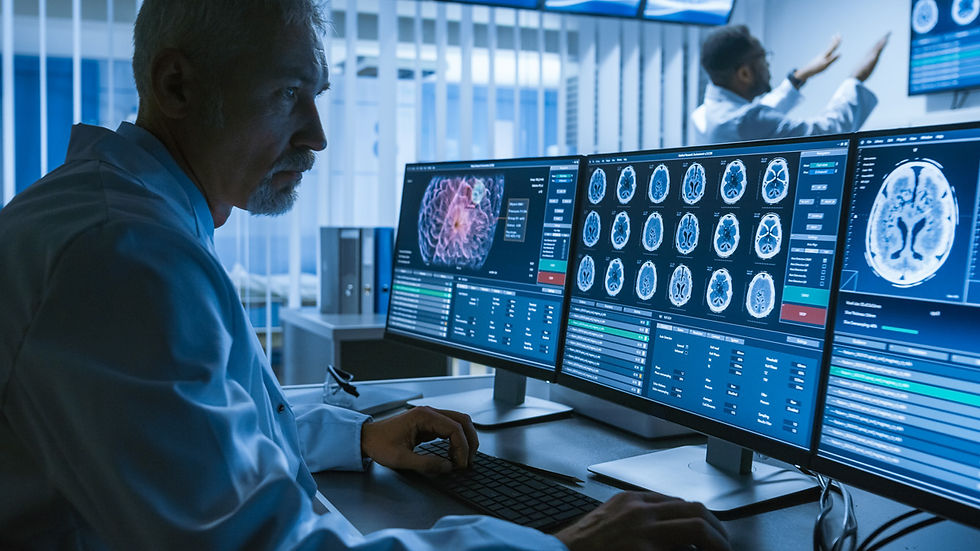
Introduction
Cancer remains one of the leading causes of morbidity and mortality worldwide, amassing a death toll of over 10 million annually (Joel S. Brown, et al., 2023). Although traditional methods of cancer research, diagnosis, and treatment are effective and are constantly improving, they often face limitations regarding speed, accuracy, and scalability (X. Liu, H. Jiang, and X. Wang, 2024). The advent of artificial intelligence (AI) offers promising new avenues for tackling these challenges. By leveraging advanced computational techniques, AI has the potential to revolutionize various aspects of cancer research, from early detection to personalized treatment strategies.
Early Detection and Diagnosis: Medical Imaging Analysis
AI algorithms, particularly those based on deep learning, have shown remarkable proficiency in analyzing medical images. Techniques such as convolutional neural networks (CNNs) can be trained to detect subtle patterns in radiographic images, magnetic resonance imaging (MRI), and computed tomography (CT) scans that may be indicative of cancerous growth (Weerarathna, I.N., Kamble, A.R. and Luharia, A., 2023). This capability can lead to earlier detection of cancers, such as breast, lung, and skin cancers, significantly improving patient outcomes.
Biomarker Discovery
AI can also aid in the identification of biomarkers—biological molecules indicative of the presence of cancer. Deep learning algorithms can analyze vast datasets from genomic, proteomic, and metabolomic studies to pinpoint potential biomarkers. This process, which would be exceedingly time-consuming and complex through manual analysis, becomes feasible and efficient with AI, paving the way for more accurate and early cancer detection (Perez-Lopez, et al., 2024).
Personalized Treatment: Genomic Data Analysis
The field of oncology is increasingly moving towards personalized medicine, where treatments are tailored to the genetic makeup of individual tumours. AI can process and interpret genomic data to identify mutations and alterations specific to a patient’s cancer. By integrating this information with clinical data, AI can help devise personalized treatment plans that target the unique characteristics of each tumour, thereby enhancing treatment efficacy and minimizing adverse effects (Perez-Lopez, et al., 2024) (Weerarathna, I.N., Kamble, A.R. and Luharia, A., 2023).
Enhancing Research and Clinical Trials: Predictive Modeling
AI can build predictive models that forecast cancer progression and patient outcomes based on historical data. These models can help researchers understand the potential impacts of different treatment strategies and identify which patients are most likely to benefit from specific interventions. In clinical trials, AI can optimize trial design by identifying suitable patient cohorts, predicting patient responses, and monitoring adverse effects in real-time (Weerarathna, I.N., Kamble, A.R. and Luharia, A., 2023) (Zhao, Y., Kosorok, M.R. and Zeng, D., 2009).
Conclusion
AI holds tremendous potential to transform cancer research, offering new tools for early detection, personalised treatment, and efficient drug discovery. By overcoming current limitations, AI can play a pivotal role in advancing our understanding and management of cancer, ultimately improving patient outcomes and saving lives. It is a general consensus nowadays that for researchers, it is important to be able to understand, interpret and critically evaluate the AI output as it will be beneficial to acquire a deeper understanding of AI and develop their own AI-based software tools. Continued collaboration and innovation between AI researchers and oncologists will be essential to fully reap the benefits of AI in the fight against cancer.
Article prepared by: Melisa Wong Siang Ming, MBIOS R&D Associate 23/24
If you enjoyed this article, do sign up to become a part of our MBIOS family and receive our monthly newsletter along with many more resources in the link below.
References
Joel S. Brown, et al. (2023) Updating the Definition of Cancer. AACR. [Online] Available from: doi.org/10.1158/1541-7786.MCR-23-0411.
Liu, X., Jiang, H. & Wang, X. (2024) Advances in Cancer Research: Current and Future Diagnostic and Therapeutic Strategies. Biosensors. [Online] 14 (2), 100. Available from: doi:10.3390/bios14020100.
Weerarathna, I.N., Kamble, A.R. & Luharia, A. (2023) Artificial Intelligence Applications for Biomedical Cancer Research: A Review. Cureus. [Online] Available from: doi:10.7759/cureus.48307.
Perez-Lopez, R., Ghaffari Laleh, N., Mahmood, F. et al. A guide to artificial intelligence for cancer researchers. Nat Rev Cancer 24, 427–441 (2024). Available from: https://doi.org/10.1038/s41568-024-00694-7
Zhao, Y., Kosorok, M.R. and Zeng, D., 2009. Reinforcement learning design for cancer clinical trials. Statistics in medicine, 28(26), pp.3294-3315.
Comentários